[Jan 25] |
Two papers accepted in ICLR 2025! |
[Dec 24] |
Participated in Immunological Genome Project (ImmGen T) consortium at Harvard Medical School. |
[Dec 24] |
Graduated with M.Sc. (Thesis) in Computer Science from McGill University (and Mila)! |
[Oct 24] |
One paper accepted in NeurIPS 2024 Workshop on RBFM. |
[Oct 24] |
One paper accepted in NeurIPS 2024 AI4Mat workshop with a spotlight talk. |
[Sep 24] |
Received EDIC Fellowship offer to start my Ph.D. at EPFL! |
[Jul 24] |
One blogpost accepted in GRaM workshop, ICML 2024! Blogpost here. |
[May 24] |
Two papers accepted in EquiVision workshop, CVPR 2024 with a spotlight talk! Slides here. |
[Apr 24] |
Gave a talk on Equivariant Adaptation of Large Pretrained Models at Google Research India. Slides here. |
[Mar 24] |
Received GREAT award from McGill University to attend ICLR 2024. |
[Mar 24] |
Released open-source Python package EquiAdapt! Feedback is welcome. |
[Jan 24] |
One paper accepted in ICLR 2024! |
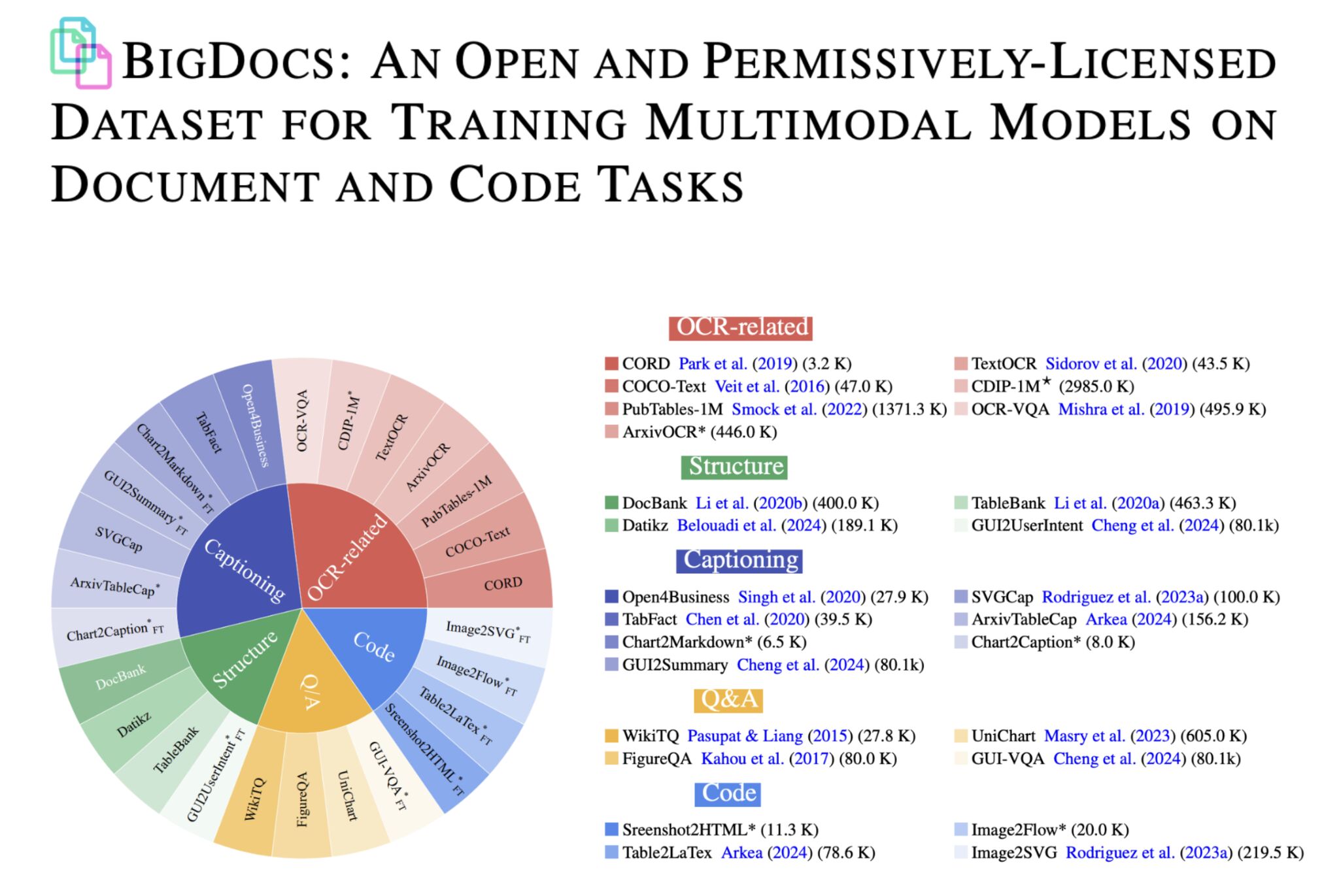 |
BigDocs: An Open and Permissively-Licensed Dataset for Training Multimodal Models on Document and Code Tasks
Juan Rodriguez*, Xiangru Jian*, Siba Smarak Panigrahi*, et al.
International Conference on Learning Representations (ICLR) 2025; RBFM workshop (NeurIPS) 2024
|
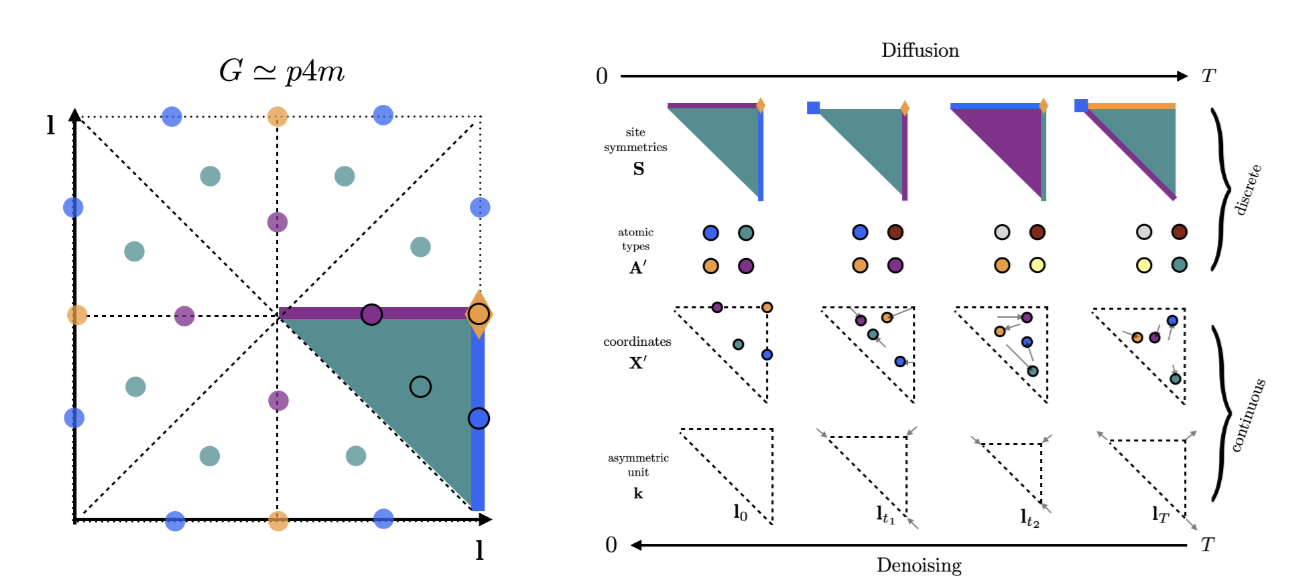 |
SymmCD: Symmetry-Preserving Crystal Generation with Diffusion Models
Daniel Levy*, Siba Smarak Panigrahi*, Sékou-Oumar Kaba*, Qiang Zhu, Mikhail Galkin, Santiago Miret, Siamak Ravanbakhsh
International Conference on Learning Representations (ICLR) 2025; AI4Mat workshop (NeurIPS) 2024 (spotlight)
|
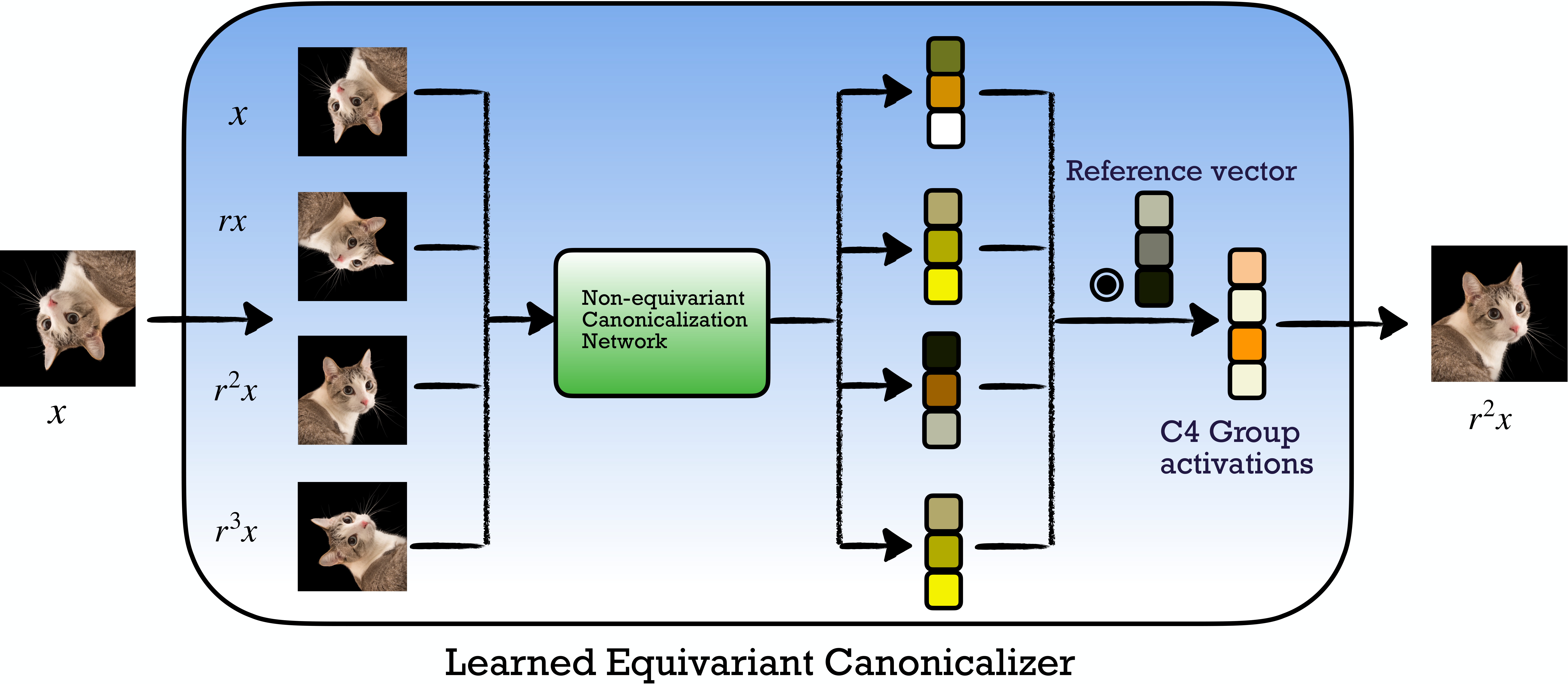 |
Improved Canonicalization for Model Agnostic Equivariance
Siba Smarak Panigrahi, Arnab Kumar Mondal
Equivariant Vision (EquiVision) workshop (CVPR) 2024
|
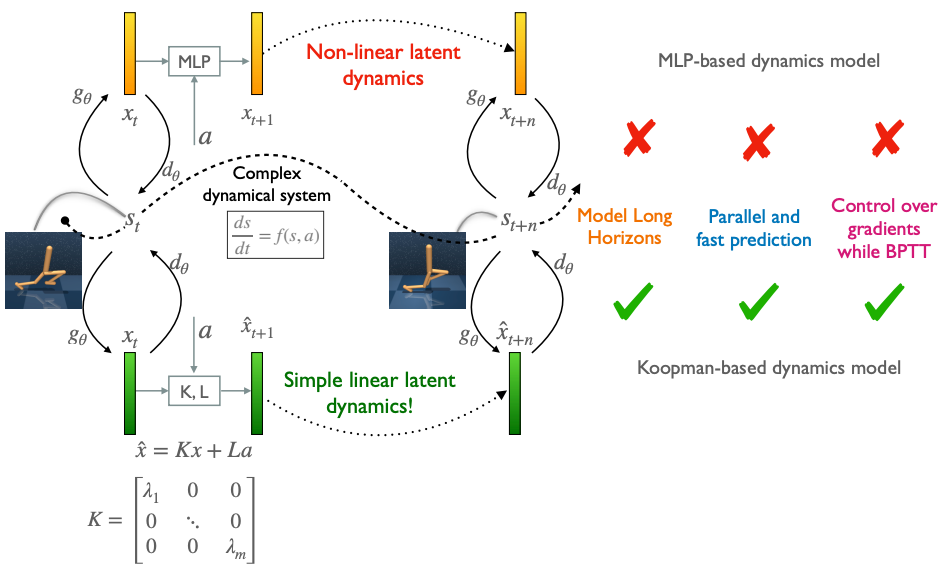 |
Efficient Dynamics Modeling in Interactive Environments with Koopman Theory
Arnab Kumar Mondal, Siba Smarak Panigrahi, Sai Rajeswar, Kaleem Siddiqi, Siamak Ravanbakhsh
International Conference on Learning Representations (ICLR) 2024;
European Workshop on Reinforcement Learning (EWRL) 2023
|
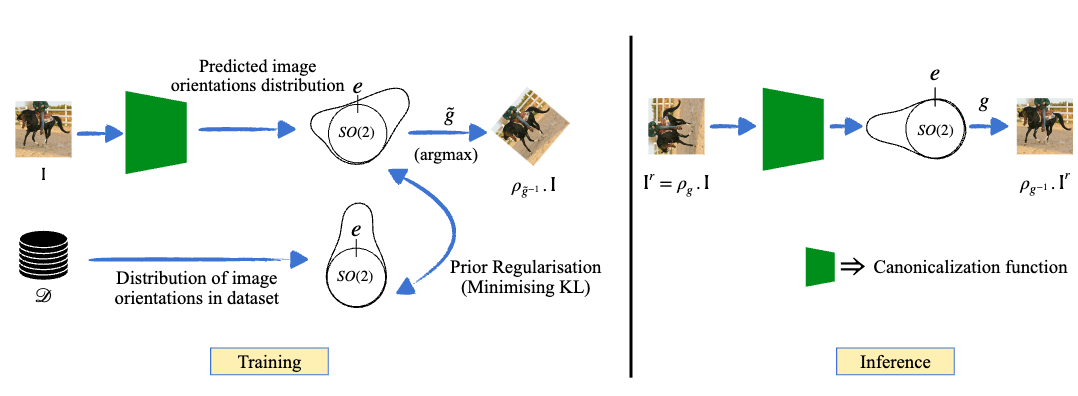 |
Equivariant Adaptation of Large Pretrained Models
Arnab Kumar Mondal*, Siba Smarak Panigrahi*, Sékou-Oumar Kaba, Sai Rajeswar, Siamak Ravanbakhsh
Conference on Neural Information Processing Systems (NeurIPS) 2023
|
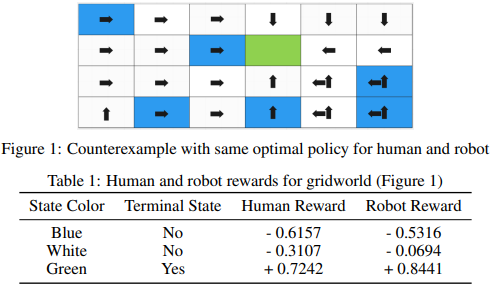 |
[Re]: Value Alignment Verification
Siba Smarak Panigrahi*, Sohan Patnaik*
The ML Reproducibility Challenge (MLRC), 2021;
NeurIPS Journal Showcase Track, 2022; NeurIPS Spotlight, 2022
|
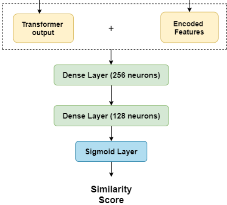 |
Leveraging Pretrained Language Models for Key Point Matching
Manav Nitin Kapadnis*, Sohan Patnaik*, Siba Smarak Panigrahi*, Varun Madhavan*, and Abhilash Nandy
8th workshop on ArgumentMining at Empirical Methods in Natural Language Processing (EMNLP), 2021
|
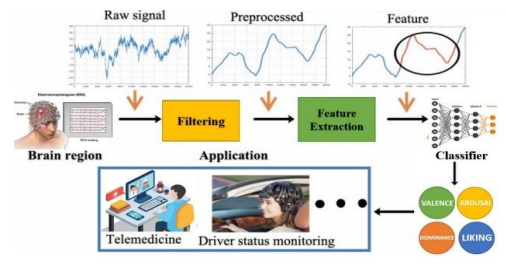 |
Multi-class Emotion Classification Using EEG Signals
Divya Acharya, Riddhi Jain, Siba Smarak Panigrahi, Rahul Sahni, Siddhi Jain, Sanika Prashant Deshmukh, and Arpit Bhardwaj
10th International Advance Computing Conference (IACC), 2020
|
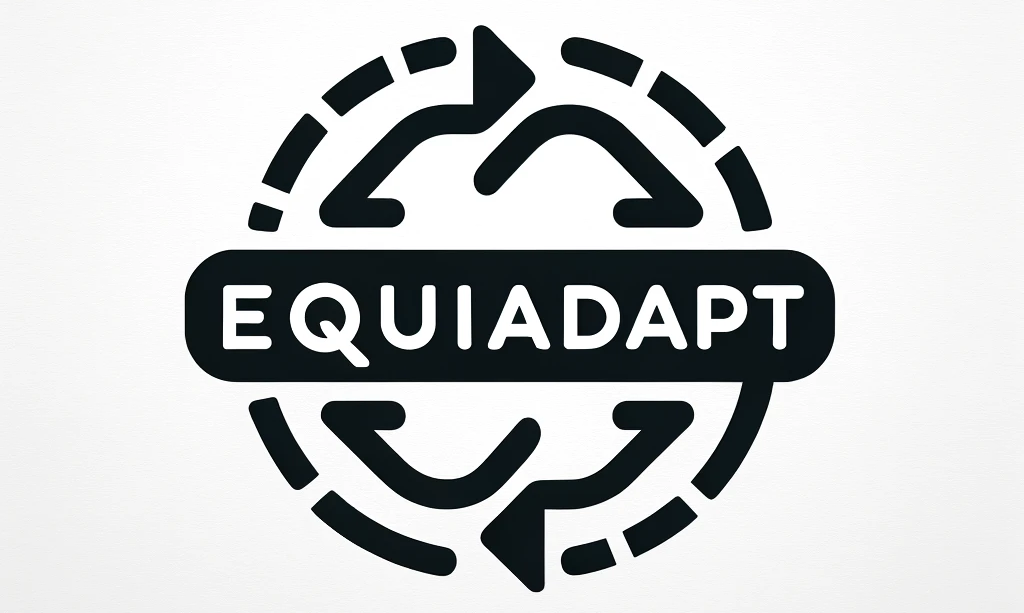 |
EquiAdapt
Library to make any existing neural network architecture equivariant.
|
|